How Does TriageGO Work?
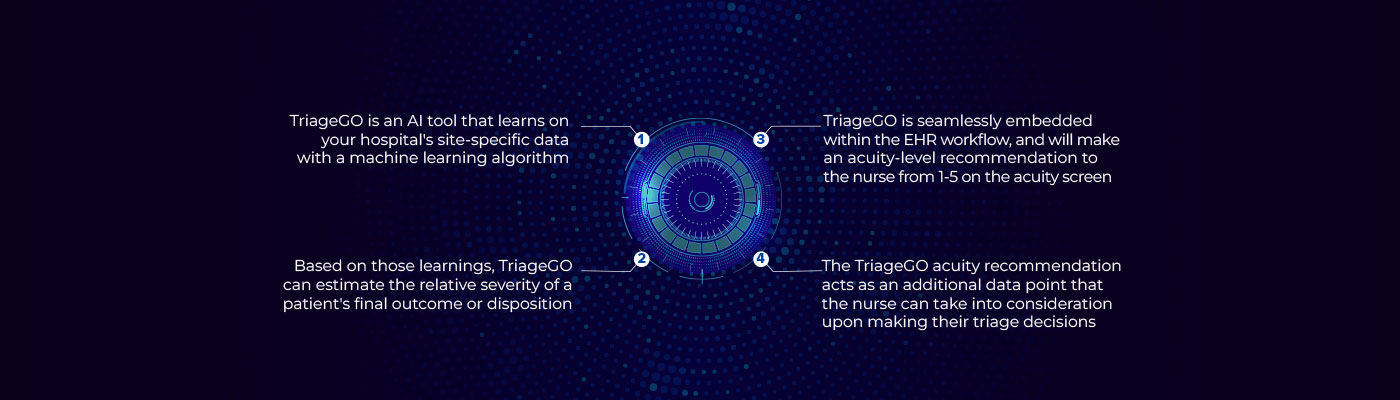
Optimized Triage with Artificial Intelligence
Unlock the power of data-driven decision making in your Emergency Department, and join the ranks of leading healthcare facilities who are transforming patient triage and empowering their nurses to deliver exceptional care.
Risk vs Resources
• Resource utilization is not a sufficient proxy to severity of illness
• Outcomes-based triage assignment outperforms resource-based triage assignment
• Decrease time to care for all patient groups, while improving operations in your ED
Saved from door to admit decision1
TriageGO is a powerful and intuitive platform that leverages artificial intelligence to provide acuity-level recommendations based on real patient data.
TriageGO empowers the nurse to assign patients to the correct areas for care quickly and accurately, streamlining the triage process and improving patient outcomes.
TriageGO improves patient flow from waiting room to provider by improving the triage process. In today’s chaotic, overcrowded emergency departments, accurate and efficient triage is vital for delivering optimal patient care.
Emergency Departments that use TriageGO can observe decreased wait-times, and decreased time-to-care for their patients3
This product is only available in the United States at this time. Please contact your Beckman Coulter sales representative or distributor for more information.
Sources:
1. Levin S, Toerper M, Hinson J, Gardner H, Henry S, McKenzie C, Whalen M, Hamrock E, Barnes S, Martinez D, Kelen G. Machine-Learning Based Electronic Triage: A Prospective Evaluation. Ann Emerg Med. 72(4), S116. https://www.annemergmed.com/article/S0196-0644(18)31035-7/fulltext
2. Internal Report: Time to Emergent Care. Data last analyzed in 2024. Aggregate of five hospital sites in United States.
3. Levin S, Toerper M, Hamrock E, Hinson J, Barnes S, Gardner H, Dugas A, Linton B, Kirsch T, Kelen G. Machine Learning-Based Triage More Accurately Differentiates Patients with Respect to Clinical Outcomes Compared to the Emergency Severity Index. Ann Emerg Med. 71(5):565-574, 2018. https://pubmed.ncbi.nlm.nih.gov/28888332/
Information addressed to health professionals. The products presented on this site are for professional use only, and, where applicable, comply with the requirements of the IVD Directive 98/79 / EC. Instructions for use must be consulted before using these products.
© 2024 Beckman Coulter, Inc. All rights reserved. Beckman Coulter, the stylized logo, and the Beckman Coulter product and service marks mentioned herein are trademarks or registered trademarks of Beckman Coulter, Inc. in the United States and other countries.
U.S. Government Users Restricted Rights - As per GSA ADP Schedule Contract with Beckman Coulter, Inc., the use, duplication or disclosure is restricted as follows: Beckman Coulter hereby authorizes user to copy those documents published on the World Wide Web by Beckman Coulter, for noncommercial use, and only within the user's organization. Except as expressly provided above, nothing contained herein shall be construed as granting any license or right under any Beckman Coulter copyright. Nothing contained herein shall be construed as granting by implication, estoppel or otherwise any license or right under any patent or trademark of Beckman Coulter or any third party.